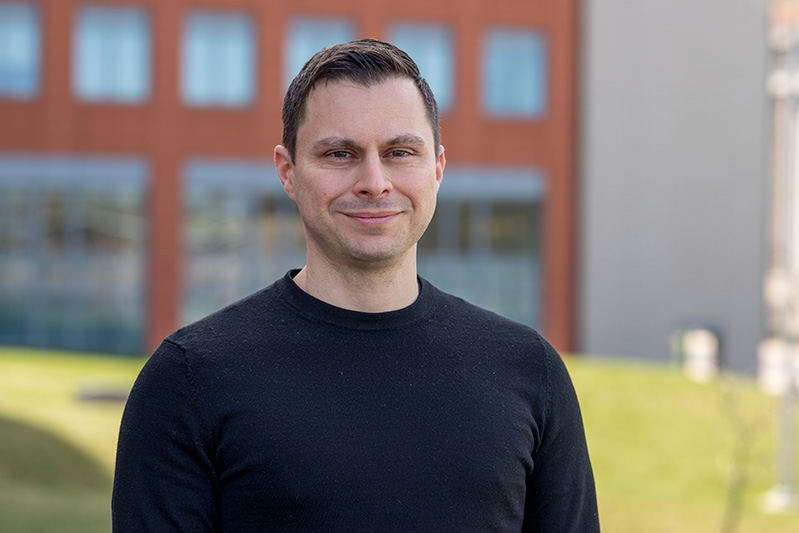
Electrical engineering and computer science Professor Ferdinando Fioretto and his research team received the 2022 Caspar Bowden PET Award for Outstanding Research in Privacy Enhancing Technologies for their paper “Decision Making with Differential Privacy under the Fairness Lens.” The award was presented at the annual Proceedings on Privacy Enhancing Technologies Symposium.
The Caspar Bowden PET award is presented annually to researchers whose work makes an outstanding contribution to the theory, design, implementation, or deployment of privacy enhancing technology. The judges said Fioretto’s team received the award for advancing the understanding of differential privacy and fairness trade-offs in decision making, providing a theoretical framework and exploring a highly relevant practical problem.
“I am honored for our work to receive this prestigious award which recognizes influential research in privacy-enhancing technologies, especially for a project that means so much to me and my group,” said Fioretto.
The awarded paper was published in the International Joint Conference of Artificial Intelligence (IJCAI) in 2021. It looks at the role of a privacy-enhancing technology (called differential privacy) in the context of Census data release for decision tasks with profound societal benefits. Some of these benefits may be the allocation of funds and resources, the distribution of therapeutics, or the assignment of congressional seats. Fioretto’s research team showed that differential privacy may induce or exacerbate biases and unfairness in many classes of decision processes and proposed a theoretical framework to audit and bound these fairness impacts.
“I am very honored and humbled to receive this prestigious award. This is one of my favorite projects and it involved a lot of hard work. Our results suggest that the US government might need to consider ethical consequences when applying differential privacy techniques to protect our privacy,” said doctoral student Cuong Tran, who was one of the authors of the paper. “I am also grateful to my advisor, collaborators, friends and staff from the electrical engineering and computer science department for helping us push this work into fruition.”
One of the main contributions of their work was to examine the roots of the induced unfairness as well as proposing guidelines to mitigate the negative fairness effects of the decision problems studied.
“I am also happy to see that the analysis proposed in our work has inspired a line of follow-up works in the field of privacy-preserving machine learning to understand why private machine learning algorithms may induce or exacerbate disparate impacts,” said Fioretto. “We are continuing our efforts in this area and are currently working with policy-makers to better understand when and how our solutions may be adopted. I am very excited to see how this direction evolves and look forward to the efforts that our community will make to build better tools to address these fairness issues in privacy-preserving processes.”