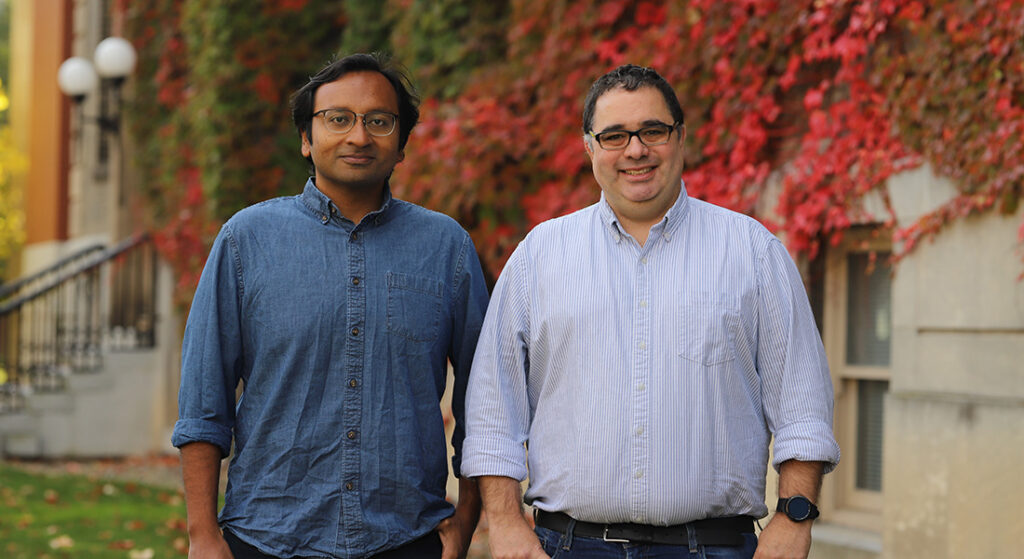
Whether you’re looking to try a new recipe, dimming the lights in your living room, or curious about the species of bacteria living inside your mouth, Amazon Alexa has got you covered. With a simple voice command, Alexa’s ability to perform various tasks or answer questions has made it widely popular, with over 40 million users in the United States alone. Despite the convenience smart speakers like Alexa offer, these devices have also raised some privacy concerns.
Amazon has been known to collect data on users which includes their shopping habits, preferences, and even their location for personalized marketing. But that’s not all. When using waking words such as “Hey Alexa” to activate smart speakers, the audio of your voice command is also recorded and stored, becoming Amazon’s property. This means that Amazon owns your voice audio and can do whatever they want with it.
“Big tech companies are using our personal information. We’re less like customers and more like their product,” says graduate student Brian Testa ’24. “I’ve always been sensitive to that. I don’t use a lot of technology at home for that reason.”
Using voice data, companies like Amazon and Google have now developed technology that poses even more threats to privacy: AI and machine learning that can determine people’s emotional state or mood from their voice. This patented technology can even pick up on feelings from emotionally neutral phrases like “What’s the weather?” Since there are no laws in place to prevent this, there’s no protection against it.
“In the US for the last five to 10 years, lots of researchers have been working on how they can use voice to infer emotions, mood or even mental health,” says assistant professor in electrical engineering and computer science, Asif Salekin. “In my own lab, we have previous works on tech that can infer mental disorders like depression, social anxiety, manic disorder, and even suicidal tendencies from one’s voice.”
While this technology can be useful in certain circumstances, most users, if not all, have not consented to having their emotions detected by smart speakers. These privacy concerns led Testa, Professor Salekin, graduate students Harshit Sharma ’26 and Yi Xiao 26, and undergraduate student Avery Gump ’24 to begin researching ways to protect users’ privacy from smart speakers.
“Consent is key,” Salekin says. “We’d still like to use smart speakers since they’re quite useful – I have them in my own home. This project was about finding a way to use these devices without giving companies the power to exploit us.”
Led by Testa, the group conducted extensive research and developed a device that can be attached to a smart speaker or downloaded as software onto a laptop. This device emits a mild noise that only the smart speaker can hear and masks the emotional tone in your voice, providing a new level of privacy protection for concerned users.
“Through the use of a speech emotion recognition (SER) classifier, a smart speaker can analyze how people are feeling based on how they sound. We created a microphone device that listens for the wake word ‘Hey Alexa’”, Testa says. “When the smart speaker activates, our device activates too and begins to emit a noise that disrupts the smart speaker from detecting your emotions. However, only the smart speaker hears this noise.”
Currently, their device masks your emotional state by presenting it as a completely different emotion. When you speak, the smart speaker may detect from your voice that you’re sad, angry, or frustrated when you’re not feeling any of these emotions. This unpredictability makes it difficult for smart speakers to accurately determine your true emotions or mood and also prevents machine learning from picking up on any patterns and mood correlations. The group hopes to improve the device’s functionality by making it mask your emotions as neutral rather than presenting them as a different emotion.
“To create the mild noise our device emits, we utilized genetic programming to identify a combination of specific frequencies that disrupt the smart speaker from determining a person’s mood,” Salekin says. “Only the speaker hears this noise, but it can hear your speech commands clearly, so the utility of the smart speaker remains intact.”
Though the sound is only detected by the smart speaker, the group wanted to see how loud it would be when the device is used. Testa played the sound in the lab when Professor Salekin was having a meeting and Salekin didn’t even realize it was playing, which showed that the noise wasn’t disruptive. Additionally, they also conducted a survey with others to see if the noise was loud enough to be disruptive.
Testa, Salekin, Sharma, Xiao, and Gump are currently working on patent submissions, form factors, and speaking with companies about commercializing their device. What sets their patent apart from similar concepts is that while past technology focused on determining people’s moods or emotions, their technology is all about protecting them. This unique approach makes their device the first of its kind.
“It was a fun project,” Testa says. “This paper was published by me and as the first listed author, I’m excited about it. I’ve been working towards my Ph.D., and this is another step towards that goal.”
“Working with the students in real-world applications and research with real results was exciting,” Salekin says. “This research has many components and the collaboration between us was great. We’re excited to see what the future for this tech holds.”