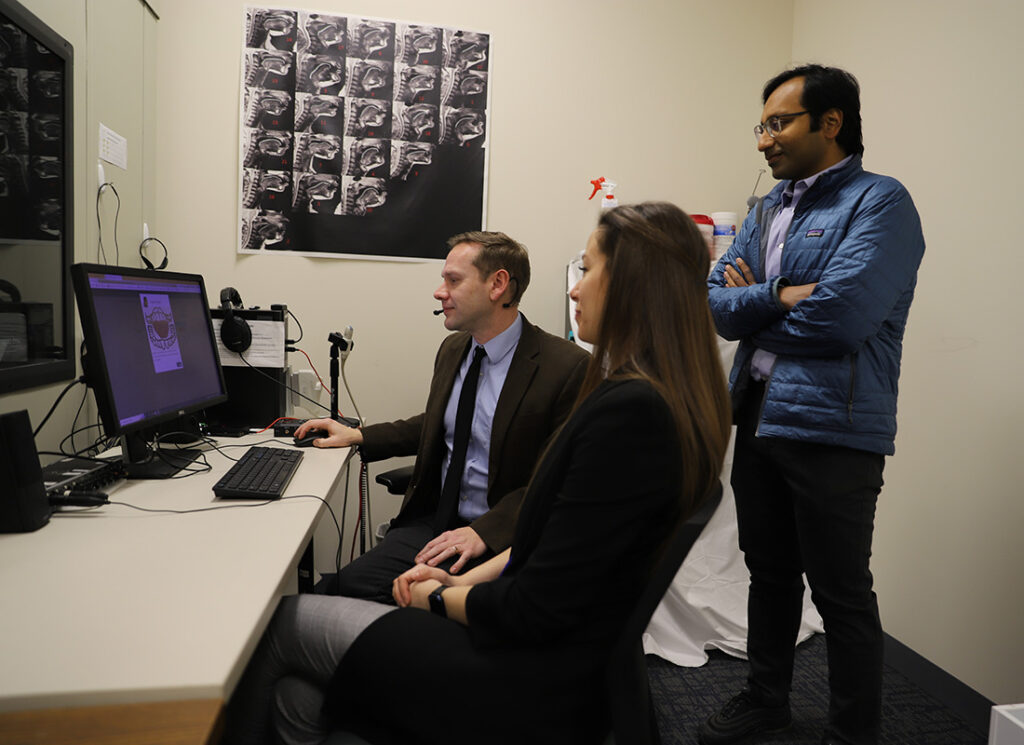
Three Syracuse University researchers, supported by a recent $2.5 million grant from the National Institutes of Health, are working to refine a clinically intuitive automated system that may improve treatment for speech sound disorders while alleviating the impact of a worldwide shortage of speech-language clinicians.
The project, “Intensive Speech Motor Chaining Treatment and Artificial Intelligence Integration for Residual Speech Sound Disorders,” is funded for five years. Jonathan Preston, associate professor of communication sciences and disorders, is principal investigator. Preston is the inventor of Speech Motor Chaining, a treatment approach for individuals with speech sound disorders. Co-principal investigators are Asif Salekin, assistant professor of electrical engineering and computer science, whose expertise is creating interpretable and fair human-centric artificial intelligence-based systems, and Nina Benway, a recent graduate of the communication sciences and disorders/speech-language pathology doctoral program.
Their system uses the evidence-based Speech Motor Chaining software, an extensive library of speech sounds and artificial intelligence to “think” and “hear” the way a speech-language clinician does.
The project focuses on the most effective scheduling of Speech Motor Chaining sessions for children with speech sound disorders and also examines whether artificial intelligence can enhance Speech Motor Chaining—a topic Benway explored in her dissertation. The work is a collaboration between Salekin’s Laboratory for Ubiquitous and Intelligent Sensing in the College of Engineering and Computer Science and Preston’s Speech Production Lab in the College of Arts and Sciences.
Clinical Need
In speech therapy, learners usually meet with a clinician one-on-one to practice speech sounds and receive feedback. If the artificial intelligence version of Speech Motor Chaining (“ChainingAI”) accurately replicates a clinician’s judgment, it could help learners get high-quality practice on their own between clinician sessions. That could help them achieve the intensity of practice that best helps overcome a speech disorder.
The software is meant to supplement, not replace, the work of speech clinicians. “We know that speech therapy works, but there’s a larger issue about whether learners are getting the intensity of services that best supports speech learning,” Benway says. “This project looks at whether AI-assisted speech therapy can increase the intensity of services through at-home practice between sessions with a human clinician. The speech clinician is still in charge, providing oversight, critical assessment and training the software on which sounds to say are correct or not; the software is simply a tool in the overall arc of clinician-led treatment.”
170,000 Sounds
A library of 170,000 correctly and incorrectly pronounced “r” sounds was used to train the system. The recorded sounds were made by 400-plus children over 10 years, collected by researchers at Syracuse, Montclair and New York Universities, and filed at the Speech Production Lab.
Benway wrote ChainingAI’s patent-pending speech analysis and machine learning operating code, which converts audio from speech sounds into recognizable numeric patterns. The system was taught to predict which patterns represent “correct” or “incorrect” speech. Predictions can be customized to individuals’ speech patterns.
During speech practice, the code works in real time with Preston’s Speech Motor Chaining website to sense, sort and interpret patterns in speech audio to “hear” whether a sound is made correctly. The software provides audio feedback (announcing “correct” or “not quite”), offers tongue-position reminders and tongue-shape animations to reinforce proper pronunciation, then selects the next practice word based on whether or not the child is ready to increase word difficulty.
Early Promise
The system shows greater potential than prior systems that have been developed to detect speech sound errors, according to the researchers.
Until now, Preston says, automated systems have not been accurate enough to provide much clinical value. This study overcomes issues that hindered previous efforts: Its example residual speech sound disorder audio dataset is larger; it more accurately recognizes incorrect sounds; and clinical trials are assessing therapeutic benefit.
“There has not been a clinical therapy system that has explicitly used AI machine learning to recognize correct and distorted “r” sounds for learners with residual speech sound disorders,” Preston says. “The data collected so far shows this system is performing well in relation to what a human clinician would say in the same circumstances and that learners are improving speech sounds after using ChainingAI.”
So Far, Just ‘R’
The experiment is currently focused on the “r” sound, the most common speech error persisting into adolescence and adulthood, and only on American English. Eventually, the researchers hope to expand software functionality to “s” and “z” sounds, different English dialects and other languages.
Ethical AI
The researchers have considered ethical aspects of AI throughout the initiative. “We’ve made sure that ethical oversight was built into this system to assure fairness in the assessments the software makes,” Salekin says. “In its learning process, the model has been taught to adjust for age and sex of clients to make sure it performs fairly regardless of those factors.” Future refinements will adjust for race and ethnicity.
The team is also assessing appropriate candidates for the therapy and whether different scheduling of therapy visits (such as a boot camp experience) might help learners progress more quickly than longer-term intermittent sessions.
Ultimately, the researchers hope the software provides sound-practice sessions that are effective, accessible and of sufficient intensity to allow ChainingAI to routinely supplement in-person clinician practice time. Once expanded to include “s” and “z” sounds, the system would address 90% of residual speech sound disorders and could benefit many thousands of the estimated six million Americans who are impacted by these disorders.
Written by Diane Stirling