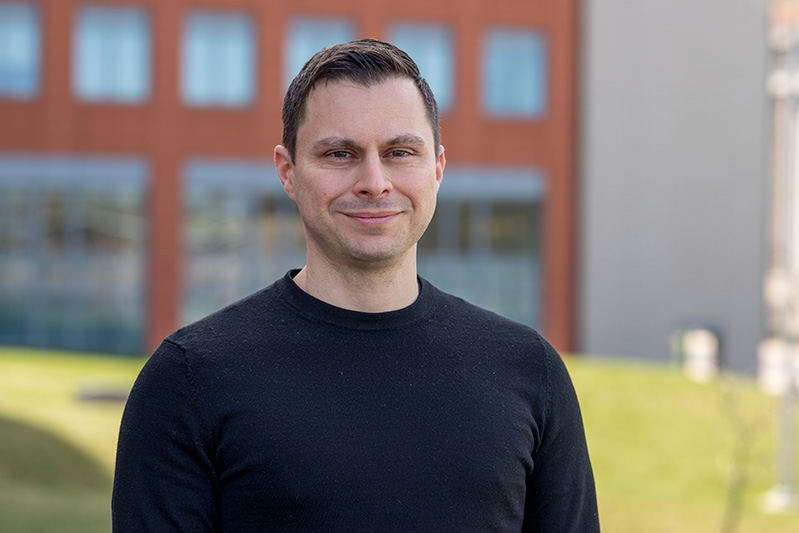
You don’t see them, but optimization algorithms are the backbone of many industrial processes that affect nearly everyone. Efficiently meeting electricity demands, matching organ donors with receivers, flight scheduling, and finding a nearby driver through ride sharing services are just a few examples of instances where complex optimization problems have far-reaching consequences. Current optimization methods are solving these issues, but they are not often finding the best possible solutions within the allowed runtimes.
“When you get sub-optimal solutions, what you lose is operational costs and societal benefits,” said electrical engineering and computer science Professor Ferdinando Fioretto. “These are all problems that are extremely hard computationally to solve, but we have to solve them in order to operate our daily lives.”
Fioretto has received an NSF CAREER Award to aid in the resolution of challenging optimization problems using machine learning. His research will primarily focus on developing a new paradigm and finding a new theoretical understanding of how to use data driven learning to accelerate the resolution of these optimization problems.
“Power systems, for example, are one of the biggest factors in climate change. If we are able to obtain solutions that make the amount of electricity that we need to produce closer to optimal, we will have substantially less waste and reduced emissions,” said Fioretto.
Fioretto and his team are focusing on discovering classes of optimization problems that can be closely approximated in near real time by integrating constrained optimization principles into the training of machine learning algorithms. Additionally, the team is attempting to gain an understanding of what classes of constraints they can effectively satisfy.
“If you have to match a rider with a driver and the driver already has a full car, that is a constraint. There is a capacity constraint that needs to be satisfied,” said Fioretto. “This is easy to satisfy when you are solving these optimizations with classical, slow, optimization solvers, but when you’re using machine learning we really need to understand how to inject this kind of knowledge into the data driven pipeline.”
To develop machine learning algorithms that satisfy constraints, this proposal will also tackle the need of generating a large quantity of high-quality data. Better data is key to figuring out what constraints can be satisfied.
“If you don’t have data then it is really difficult to solve this problem,” said Fioretto. “I am deriving new sampling processes and smart ways to reduce the amount of data to supply to a learning mechanism so that the quality of the resulting solutions can still be very high.”
NSF CAREER Awards are designed to support early-career faculty who can serve as role models in research and lead advances in the mission of their department. A limited number of awards are given each year and Fioretto is grateful that his work has been recognized.
“This is a big recognition from my research community, especially in this early stage of my career. In a sense, it is a validation and tells me that what we are doing as a group here at Syracuse University is important and well appreciated,” said Fioretto.
In addition to his NSF CAREER Award, Fioretto was recently awarded a Google Research Scholar award. The award provides an unrestricted gift to support research at institutions around the world and is focused on funding world-class research conducted by early-career professors.
“Whenever you get that validation, by a newly accepted paper at a top-tier conference, a new grant, or by receiving an award, that is a big motivation for continuing and stepping up with your work,” said Fioretto.
“Dr. Fioretto’s research embodies so much of what we are determined to achieve at Syracuse University. These optimization problems are phenomenally difficult to solve, but we cannot turn away from them simply because of their difficulty,” said J. Cole Smith, Dean, College of Engineering and Computer Science. “Using fundamentally new methods at the intersection of machine learning and optimization is an exciting bold step in his field, and those steps are going to be necessary to achieve transformative results. Moreover, the problems he examines are those having profound societal impact. Dr. Fioretto’s research vision – and his demonstrated dedication to students – is worthy of prestigious awards, and I am thrilled to see his success.”